AI and machine learning in fuel retail: transparency first
As we’ve noted time and again, once a thing of science fiction, artificial intelligence is now a real and integral piece of human life.
As AI, and the underlying machine learning methods that create it, continue to reshape the way consumers live, think, and buy, businesses need to understand the new advancements in this field, their limitations and their business applications; and then decide how they’re going to respond. This is especially true in a market like fuel and convenience retailing which is a niche industry marked by high-impact fluctuations within the market.
As the complexity of market data increases within our industry, the complexity of the algorithms you use to process that data must also increase. There are a number of complex and varied machine learning techniques out there, and using these correctly depends on understanding which of them are applicable to which problem. Therefore, context is everything.
Let’s dive into the “what and how” of using machine learning models for fuel retail.
Transparency for Trust
At one end of the spectrum are very simple and highly transparent methods for understanding the relationship between two factors, e.g. price and volume, or number of pumps and sales. At the other end of the spectrum are ‘black-box’ methods that model complex relationships but provide no visibility or explanation around these relationships, much like the human brain. This black-box style of deep learning neural networks is not necessarily the most conducive to success in day-to-day fuel retail strategy or execution. Indeed, CEOs overwhelmingly agree that AI-based decisions need to be explainable in order to be trusted. And trust is paramount for successful execution.
This is why, in order to execute reactive pricing tactics, simply employing a deep learning model is not the ideal. These models have their place in understanding longer-term demand patterns and modeling to set overall goals and strategy, but this black box won’t allow you to see why each variable impacts each possible tactical outcome.
You can certainly explain most decisions your brain makes, but not necessarily the patterns or paths it followed to reach those decisions — and you’re rarely asked to. But when learning happens via technology, in a place you can’t see, with information you can’t ever access, the decisions based on the learning’s output are liable to be called into question.
Transparency is an important attribute of useful models and central to modeling best practices, as it enables users to identify and understand the assumptions, relationships, and data used in the model. But why is this so important in fuel retailing?
To put it simply, just knowing that a given price results in higher volume is not enough. Indeed, human nature demands more of us. Why is this particular price considered “optimized?” Why is one price better than another? Why does a certain strategy work at one site, and not the other? Understanding the answers to these questions will increase confidence and trust. But knowing the “what and how” behind the model itself will also reduce the need to justify each and every move.
How can you account for all of this in the way you use technology to bolster your strategy? Choose a model that matches the demands and specifications of your industry, and don’t turn your nose up at context — you can’t make strong decisions, today, without understanding, at some base level, how the output used to make those decisions came into being.
Additionally, even knowing how to build the model (or how it will be built) is dependent on understanding the input available. Is the data clean enough? Is it the right data for the model you want to build? Is the model, itself, going to be fit to a purpose?
What’s Next?
AI experts have made great strides in the past decade. Few can predict the future, but if the industry continues to develop at the same rate, then the outlook for AI and machine learning is certainly promising. Computers will almost certainly become more personal, leading to better, faster, and stronger innovations.
Businesses and consumers alike will need to keep pace with these innovations as they come. Ultimately, AI is likely to have a significant impact on the way you operate your fuel retail business — but transparency should always remain part of your strategic approach. Even later down the line, when a black-box approach could become a necessity of complex data and technologies, your fuel retail pricing tactics should be relatively easy to trace back to their roots. Want to learn more about how you can get started with new technologies, sooner?
Read more articles about:
UncategorizedSubscribe and get the latest updates
You may unsubscribe from our mailing list at any time. To understand how and why we process your data, please see our Privacy & Cookies Policy
Related posts
Fuel pricing
Fuel Pricing AI – one size doesn’t fit all
Despite the obvious plus points of AI, it is not the ideal solution for everyone. Many fuel retailers are hesitant to...
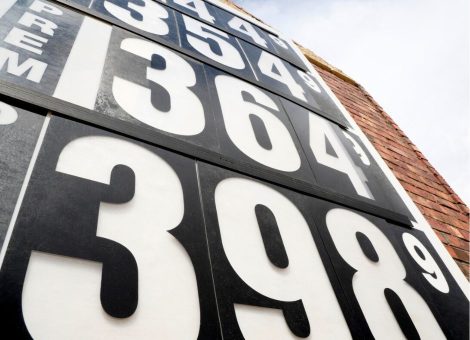
Meet the Kalibrate team
Delivering the Kalibrate Standard: Riley Buchanan
This blog series explores how members of our team help our clients answer their most challenging questions. Here, we...
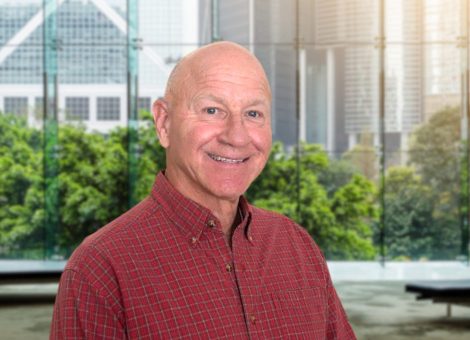