AI series part 3: How much should you rely on artificial intelligence in fuel pricing?
In other words, if you have access to copious amounts of data and a variety of machine learning models and automated responses to work with, it’s very easy to fall into a data/pricing spiral. Every hour, you receive new data, so every hour you make new changes. This practice is not only unwise — it can be perilous. Why? As you make more changes, you can create more volatility, which ultimately will reduce your margins. As your market matures, volatility is already inevitable. Contributing to that volatility at a pace quicker than necessary is detrimental to your overall success.
If you begin a foray into intra-day pricing with the intention of increasing profits, but without a full understanding of how your customers or your competitors will react, you will probably see the opposite impact on profitability. The interplay between you, your competitors and your customers’ reaction can create a pricing death spiral from which it is difficult to resurface. With all that said, if your market is already committed to intra-day pricing, you must, of course, keep up with a well thought-out strategy. But if you’re in a stable market, you don’t want to be the competitor who forges ahead reacting to every piece of data you receive. By all means, if you want to be a disruptor in the market, and test out different strategies and positions in order to understand the impact of these “disruptive” price moves, then do so using a controlled, measured and strategic approach.
Think of it this way: Let’s say you bought a Ferrari that can go faster than a dream. You don’t need to go 120 mph at all times, despite the fact that you can. If you go 120 mph on that road that’s designed for only 25 mph speeds, with limited experience on how to handle the car, you’re going to crash!
Simply put, complete reliance on machine learning and AI can become risky, because reacting to new data immediately is not always the right thing to do. The key to success is understanding when that fast reaction is right, and when it is not. In stage 2 of maturity (unstable market), you may want to dial back your response time. Collect the data and learn from it — but don’t react immediately. In stage 3 (competitive market), the frequency of data input may change. Your system should be readied to support the frequency at which you would like to price, rather than set to make the decision around delay (or not) for you. After all, the system itself is likely unaware of the market maturity phase you are entering or already in.
When you’ve entered stage 3, then, what is the right way around reliance on machine learning when you are trying to optimize pricing efficiency? Consider the Ferrari example again — your car can be as fast as you want it to be, but you need to know how to drive it, and where you’re driving it. Expertise and context are both required in order to get the most from your vehicle and still avoid crashing. That’s why your fuel pricing needs go beyond “the system.” You also need advice. This can go a long way toward utilizing the system’s full potential — and your full potential as a strategist, too.
We mentioned previously that you can also gain experience and context via testing. You might select certain sites where you would like to be more aggressive on price. But be aware that this aggressiveness does not create a domino effect, trickling out of your one, experimental site and spreading to a much larger area. If you plan to test, you must do so in a controlled environment — dictate your boundaries, and elucidate the entire strategy prior to enacting any changes. Bottom line: If you want to gain experience and context through running a test that might promote volatility, you need to have a clear stopping point.
As you attempt to find the right balance of pricing frequency and data input for your market, it’s important that you navigate your way through market maturation. Recognizing the change or inflection point at which it makes sense to transition toward intra-day pricing without causing volatility is a science and an art. If you don’t have decades of experience in watching markets evolve, you will either be behind the curve (not transitioning to intra-day quickly enough) or ahead of the curve (creating volatility through encouraging competitors to price often). Our best suggestion? Work with a partner, like Kalibrate, to determine the right pricing strategy for your market — whichever phase it’s in.
Read more articles about:
UncategorizedSubscribe and get the latest updates
You may unsubscribe from our mailing list at any time. To understand how and why we process your data, please see our Privacy & Cookies Policy
Related blogs
Uncategorized
AI series part one: How artificial intelligence and machine learning have advanced with data proliferation
In our three-part series on artificial intelligence and machine learning, we'll help you understand the differences...
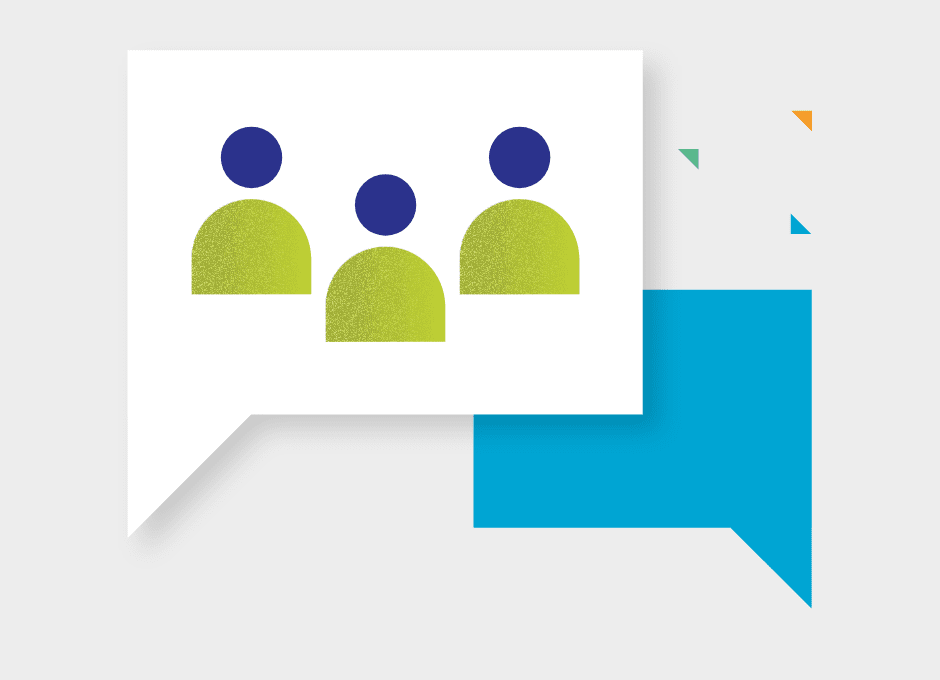
Uncategorized
AI series part two: How artificial intelligence and machine learning fit into your fuel pricing strategy
As machine learning becomes more accessible for fuel retail teams, pricing strategies and processes will inevitably...
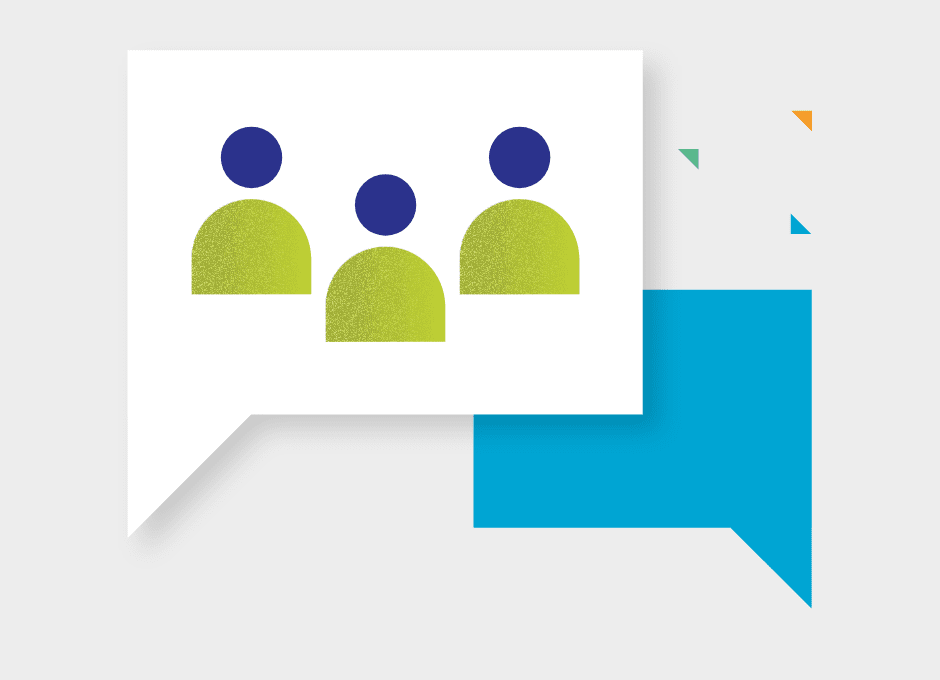