How well are you treating your data?
These days, within fuel retailing, most analysts’ primary challenge is not a lack of data, but often, too much of it. We can become swamped with the massive amount of data that is generated on a daily basis, and so the question becomes: What do we do with it? How do we use all of that data to make successful, proactive decisions about our fuel retail network?
As we’ve said many times, dynamic predictive modeling with complex machine learning algorithms is key to managing, analyzing, and visualizing fuel pricing and network planning data successfully. But as these tools become more complex, they also become more data hungry. They rely on the data that is fed to them to provide actionable insight — and if you don’t feed them good, clean, treated data, the information they spit back at you won’t be insight. It will be noise.
That’s where data treatment comes in. Data treatment, the process of preparing and filtering the data that you feed into your algorithms, should be the first step in data modeling. Efficient and effective data treatment is essential for making the best use of the data you have available to build complex models that will enable you to draw accurate conclusions. Unfortunately, the importance of the sophistication needed for good data treatment is often underestimated.
Whether you’re going to use data for analysis or decision making, you need to treat the data first — or else risk squandering your investment in both technology and effort.
The Implications of Data Treatment in Your Fuel Retail Network
In the fuel and convenience retail industry, data is extremely dynamic, constantly updating. Depending on the market, fuel prices might be updated ten times a day, and if it’s feeding into your ongoing decision making, that data needs to be treated instantly.
Most analysts have always recognized that raw data will come with some anomalies. For example, if your site has no sales because of a snow storm, basic anomaly detection can extract that outlier for you. But as more complex and dynamic data is made available, you have to be smarter about using those anomalous patterns to understand how to build data models that account for them in the first place. The algorithm needs to know not only when to take anomalous data out, but also when to put imputed data in to fill the gaps.
No matter what data you use or how complex the model, untreated data could have serious implications on the accuracy of your retail network decisions. For example, if you’re using data to build a predictive model, to be used by a price optimization tool, the level of data “missingness” may mean you need to collect much more data before you can build an accurate model, or too much noise in the data will lead to sub-optimal price generation as a result of inaccurate forecasting. If you’re building a network planning model, you need to be able to model the impact of each variable in your network, and account for ad-hoc missing data rather than just discarding anything that is incomplete.
Mind you, “fixing” your data in this way doesn’t mean manipulating it to give you the results you want. On the contrary, data treatment is all about making sure that you have a set of data that will allow you to produce the best, most accurate models possible. And you don’t require terabytes of data in order to do this. You simply need to understand what kind of data applies to your specific retail network, which pieces are more important than others, and how it will impact your decisions — that’s the data you clean and model.
Clean, Purpose-Built Data Modeling for Real-World Application
Data treatment is an ongoing process; you don’t just clean the data once and then continue to feed your model new, raw, unclean data, or you’ll be right back at square one. The process of data treatment needs to be built into the model learning from the start. So how do you build a model with effective data treatment for your retail network?
Simply running descriptive statistics to explore the data and then applying simple methods to impute or delete data points isn’t enough. Data treatment in itself requires complex modeling techniques, and you need someone with the domain knowledge and experience to understand when the treatment is right for your business and when the data is ready for modeling. Only an expert who understands both the implications of missing data and the implications of filling in incorrect data can build a model that is statistically accurate.
The best researchers will take an open-ended approach to investigating and understanding the data needed to build an effective model. If there’s new data or variables that factor into your fuel pricing strategy, such as weather, traffic, or economics, partnering with someone who can research those variables and understand the relationships is critical. Some of this can be automated, some of it can’t — and part of that expertise is knowing the balance between automated and manual data processing.
Elevate Strategy with Clean Data
Collecting, compiling, and analyzing good data can make the difference between success and failure in your fuel retail network. But in order to build accurate, dynamic predictive models that can elevate your fuel network strategy, you need to place as much importance on data treatment as data modeling.
Based on our experience with different types of markets globally, Kalibrate is a leading expert in data treatment and modeling. Interested in learning more about how we can help you achieve fuel retail success? Request to speak with one of our strategy specialists today.
Read more articles about:
UncategorizedSubscribe and get the latest updates
You may unsubscribe from our mailing list at any time. To understand how and why we process your data, please see our Privacy & Cookies Policy
Related posts
Location intelligence
Can you forecast cannibalization when opening new locations?
Understanding how a new store might will existing locations is critical for retailers and franchise businesses alike....
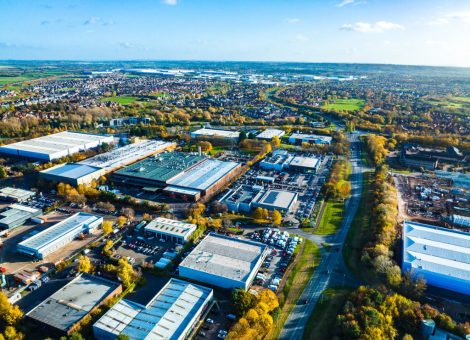
Fuel pricing
The fuel pricing maturity curve
Fuel pricing maturity is not about the size of your business – it’s about the efficiency of your fuel pricing...
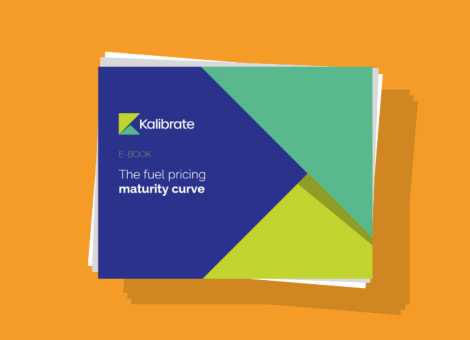