Data silos: Why they happen and how to break them down
Data silos are a common issue among companies that approach Kalibrate. Often, they are created organically as companies grow and add data, tools, teams, and components. But what are the risks associated with data that isn’t centralized? And how can organizations break down these silos?
Examples of silos include sales (POS) data in a financial system separated from leasing (expirations dates, rent, CAM charges, etc.) data. You find silos between accounting systems, customer data (including loyalty programs and delivery). Companies might hold sales and marketing data in one platform and campaigns in another. We’ve seen real estate and pipeline management in a CRM or even just Excel, integrated with no platforms that can inform the decision making, and many more.
Often silos occur simply because teams expand and need more robust tools or because acquisitions led to parallel disparate systems. Whatever the reason, data silos create inherent dangers.
Decision-making is impaired when you don’t have the complete picture and rely on guesswork to fill in the blanks. This creates risk and an increased potential for mistakes.
How confident can you be that the data you are using is the most up-to-date? Is it the same data other teams are using to make critical decisions? Silos can also put pressure on a small group of people to locate, pull, and distribute the needed data. This creates bottlenecks to accessing data within reasonable time frames. As an additional challenge, pulling and distributing data can lead to even further silos as those using the data may update and append their copies, which are not shared with others.
Incomplete data
The first step to a solution is data centralization. Organizations that do this well usually have some type of data warehouse for the most critical data, either in a private data center or behind a firewall. Doing so allows those people given the responsibility of making important, and costly, decisions to execute efficiently, with the most up-to-date data available. It also allows for teams to work from that same updated data, creating “one version of the truth”. Think about it – if real estate is opening locations using different data than marketing, how will they be able to effectively campaign for the new store opening? Similarly, if you’re asking real estate or research to determine a viable market or network optimization strategy, how effective can they be if using outdated or incomplete data about the competition or desired co-tenants?
Many companies depend on sales forecasting models to determine site viability. Good models are critical, but they can only be as good as the data that feeds them. The old adage – Garbage In = Garbage Out holds true. In recent years, we’ve seen countless retailers announce massive store closings, bankruptcy filings, and even liquidations. How many of those made real estate decisions based on analytics with outdated or incomplete data? Sure, sometimes macro and microeconomics change the outlook of a concept or brand, but store networks built using faulty data won’t succeed over the long term.
When challenges occur, lacking access to the right insight to, for example, adapt your marketing strategy or rightsize your store portfolio – or even identify what’s causing the issue in the first place – becomes impossible.
This brings us back to the realization that data is the key. Not just lots of it, but highly trusted and shared data that is updating in near-real time. Another critical component is data updated from the field. No third-party dataset stays as accurate over time as insight from your team on the ground, especially in today’s retail environment. Enabling your field teams, including real estate, broker partners, and operators, to add, verify, and enhance your data is critical – even more so if you rely on models. How many times has a model indicated the viability of a site, without knowing or calculating for the most recent competitor that has entered or announced entry into that market? Scenarios like this are a case study in why it’s crucial to get regular intelligence from field teams and centralize it within your organization.
Data and centralization: a cost-benefit perspective
It’s surprising how many organizations when making real estate decisions worth millions of dollars, resist investing in tools and data sets that inform and protect those investments. Those that do recognize the value quickly learn that quality data not only improve outcomes but also significantly supports risk management. That’s when we truly see how important the conversion from data silos to data centralization really is.
A Kalibrate approach
At its core, Kalibrate Location Intelligence is a data gathering, validation, and enablement platform. We begin with data centralization and then provide tools to continuously enhance and improve the data you rely on to make critical decisions.
From your location strategy, omnichannel approach, competitive assessments, store format, pricing, marketing, and lease renewals, silos can slow down even the nimblest organization. In today’s retail environment, that’s not a place you want to be.
To find out more, speak to a member of our team.
Read more articles about:
Location intelligenceSubscribe and get the latest updates
You may unsubscribe from our mailing list at any time. To understand how and why we process your data, please see our Privacy & Cookies Policy
Related posts
Location intelligence
Can you forecast cannibalization when opening new locations?
Understanding how a new store might will existing locations is critical for retailers and franchise businesses alike....
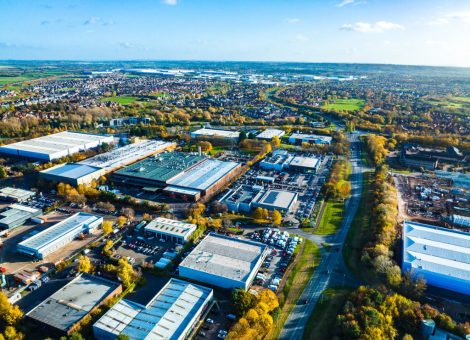
Location intelligence
Kalibrate enhances location platform with Microsoft Azure AI Foundry capabilities
The Kalibrate Location Intelligence platform integrates with Microsoft Azure AI Foundry to deliver faster,...
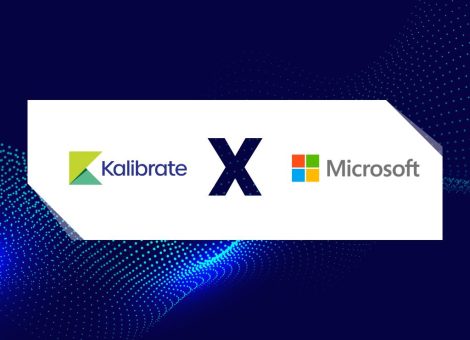