The power of fuel price data: handling "missingness"
We’ve said it time and time again, in order to thrive in today’s competitive fuel retail market, you have to take a data-driven approach. If you’re not using fuel price data to make decisions about your network, then you might as well be pushing buttons wearing a blindfold. By conducting a comprehensive competitive analysis, fuel retailers will understand:
- Who their true competitors are
- Key differentiators between their sites and their competitors’ sites
- The volume potential of each site
- Daily, weekly and monthly demand cycles at each site
- Market volatility
- … and more!
One of the most important drivers for sensitivity is relative price. It’s true that actual price has some effect on sensitivity; when gas is cheaper, people will inevitably drive more. But at a micro-level, consumers are going to make decisions based on the relative prices of different sites. Data collection of competitor prices becomes critical in any competitive market where prices are rapidly changing and the relative position vis-a-vis your competitors is a strong driving force behind your share of demand.
Shortcomings in Competitor Pricing Data
But here’s the problem: Given the market maturity and volatility of prices, gathering data on competitor prices with the right frequency can be a huge pain. To ensure complete, accurate data, network planners would have to survey multiple sites several times a day. Even if you do manage to conduct surveys with the higher frequency, it can be difficult to ensure you’re collecting data on the right competitors at the right time. There are quite a few companies who collect and sell this information to retailers, but even then, there’s no guarantee it’s complete, timely and comprehensive.
The impact is that you can’t have an appreciation for your true price position relative to your competitors, and if you don’t know that, it will be next to impossible to put together a suitable price positioning strategy. Furthermore, if you’re using incomplete data to model, forecast and optimize, you will not be able to produce effective, accurate modeling or truly-optimal prices.
So how do fuel retailers deal with this “missingness” of pricing data?
Filling the Gaps with Smart Imputation
To address this problem, you need to first understand whether or not you even have a problem. You have to ask yourself: Is my data complete or incomplete? If it’s incomplete, then you know to take it with a grain of salt. But what if you could predict and fill in those gaps appropriately, enabling you to make better, smarter decisions with that data? The good news is that this can easily be done with the right technology.
A good data analytics system will provide you with a data completeness radiator to gauge the data’s extensiveness and appropriateness to temper your expectations regarding how well subsequent modeling can perform. A great system will be able to predict the missing data and fill in the gaps with smart imputation, improving the completeness and enabling accurate analysis and modeling.
With smart imputation, it’s okay to have some level of “missingness” in your data. Gaps are inferred based on local market behavior, domain knowledge, volatility levels and other data-mined information to ensure that inferred values have similar properties to observed prices. By smartly inferring this data, you can continue to build good models and optimize your strategy effectively. Automatically minimizing data-related issues in day-to-day pricing has the added benefit of reducing support overhead as well.
Continually Optimize with Complete, Correct Data
Keep in mind: The only way to properly optimize your strategy is to analyze all of the volume magnets, not just pricing. Determine your key competitors based on their overall impact, which will result from a combination of pricing, brand, distance, attributes and more. When you understand how these things work together, you can build more holistic and effective models.
However, to build any kind of model at all, you need good data. Without it, you’ll have poor, inaccurate forecasts, and you won’t be able to optimize in the future. Be smarter about your data collection, compilation and analytics processes. Know your data shortcomings, smartly fill those gaps and optimize your strategy accordingly.
Read more articles about:
Fuel pricingSubscribe and get the latest updates
You may unsubscribe from our mailing list at any time. To understand how and why we process your data, please see our Privacy & Cookies Policy
Related posts
Location intelligence
Condado Tacos selects Kalibrate to drive growth and open new locations
Kalibrate to support the restaurant's real estate and store expansion strategy.
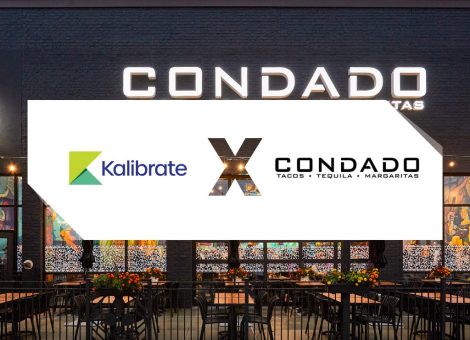
Fuel pricing
January 2025. Kalibrate's Canadian Petroleum Price Snapshot
Kalibrate conducts a daily survey of retail gasoline, diesel, propane, and furnace fuel prices in 77 Canadian cities....
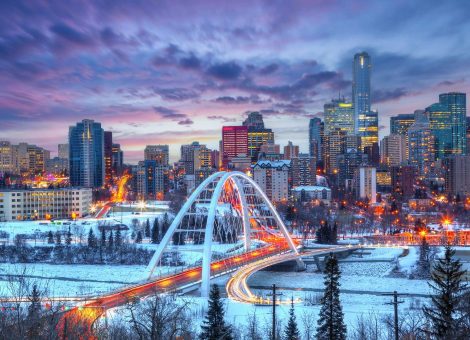